SEMINAR PROFILE
- Period
- March 8-10, 2019
- Place
- Google Japan
(Roppongi Hills, 6-10-1 Roppongi, Minato-ku, Tokyo 106-6108, Japan) - Representative Organizer
- Satoru Hashimoto (Kyoto Prefectural University of Medicine)
- Co-organizer
- Hidenobu Shigemitsu (Tokyo Medical and Dental University)
*Hands-on Workshop will be also held on March 7, 2019 at the Tokyo Medical and Dental University (MD Tower 2F) as the supplemental program.
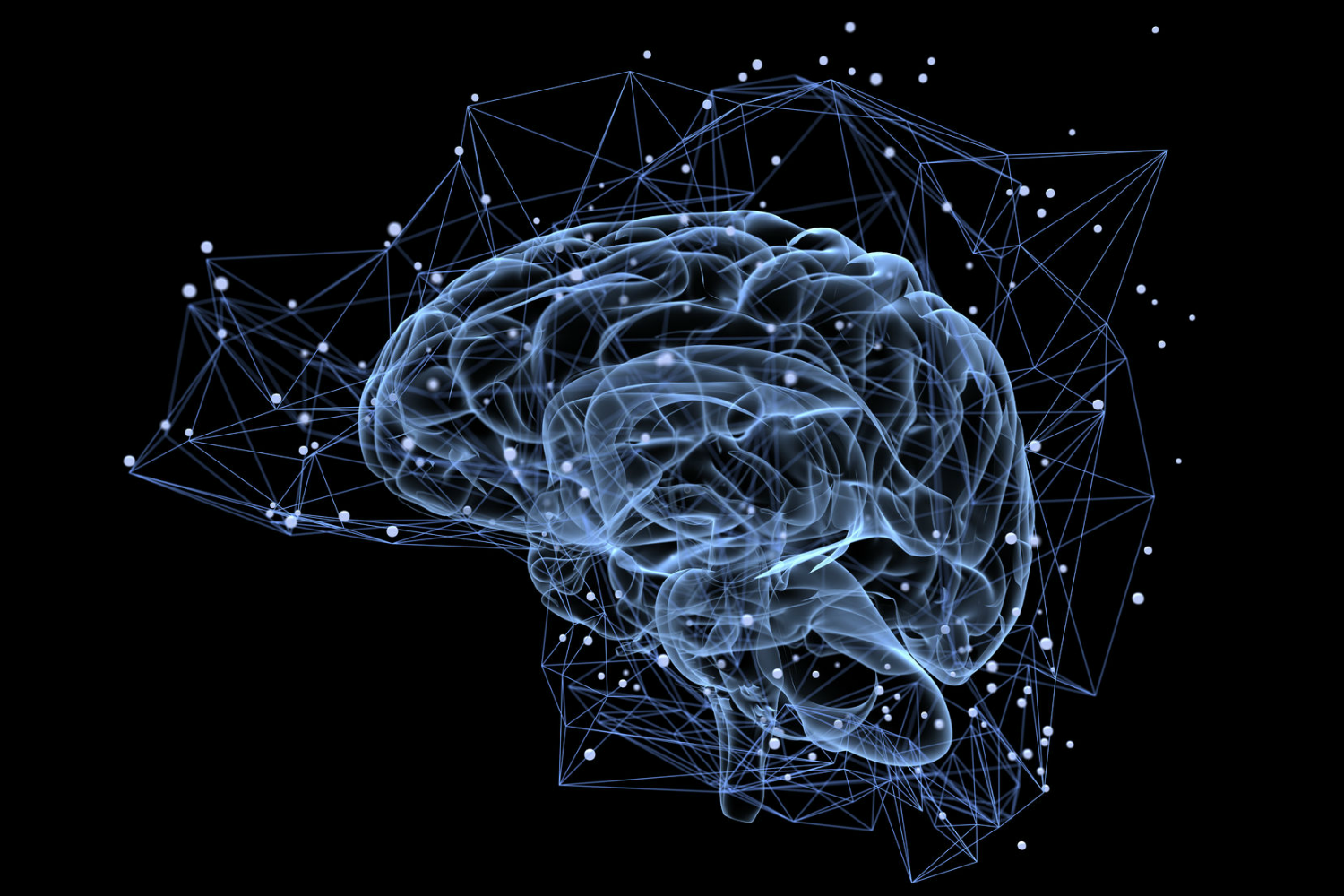